In recent years, data has dethroned oil and become the world’s most valuable resource.
Most companies understand the importance of data, soliciting customer feedback and utilizing numerous tools for analytics. Many also appear to recognize the value of experimentation as a source for great insights. However, when it comes to executing smart business experiments, they prove that it’s easier said than done.
Beyond a standalone product or website survey, A/B testing is one of the most popular ways to gather data, particularly in online settings. Many companies use A/B testing to measure the efficiency of two versions of a webpage expected to convert visitors into customers, like a landing page for a product. According to Invesp, 71 percent of companies run two or more A/B tests a month.
But what exactly is an A/B test, why is it important, and what advantages does it offer over the guessing methods many people still use?
What is A/B testing?
A/B testing is controlled experimentation that allows you to simultaneously compare “like for like” scenarios where one version has a different element. ‘Bucket’, ‘split’, or ‘split-run’ tests are other names for this type of experiment.
A/B tests involve the use of a randomly selected “control group” and a “treatment group.” The control group represents the experiment’s comparison standard. You use this set of individuals to establish the baseline measure.
In the context of webpage testing, these are the visitors you send to your current version of a given page, one with a conversion rate you’re looking to improve.
The treatment group, on the other hand, are the recipients of the experiment’s varied element. These visitors will instead be directed to the new version of the same page which you’ve come up with to test. It might have a different offer, color scheme, or call to action.
An A/B testing tool then measures each group’s engagement with the page to find out which version is the most popular. In short, the tool will tell you which page is more successful at converting leads into customers.
Since you’ve only changed one aspect of the page, you can reasonably assume that the change is responsible for any differences in conversion rates, as long as you’ve had a reasonable amount of traffic to each version.
As well as webpages, A/B testing can be run for emails, multimedia marketing strategies, paid internet advertising, and newsletters — nearly any step in the customer journey. Web design is the most popular form, though, offering so many ways to control visitors’ experience and such rich opportunities to increase traffic and conversion and lower bounce rates.
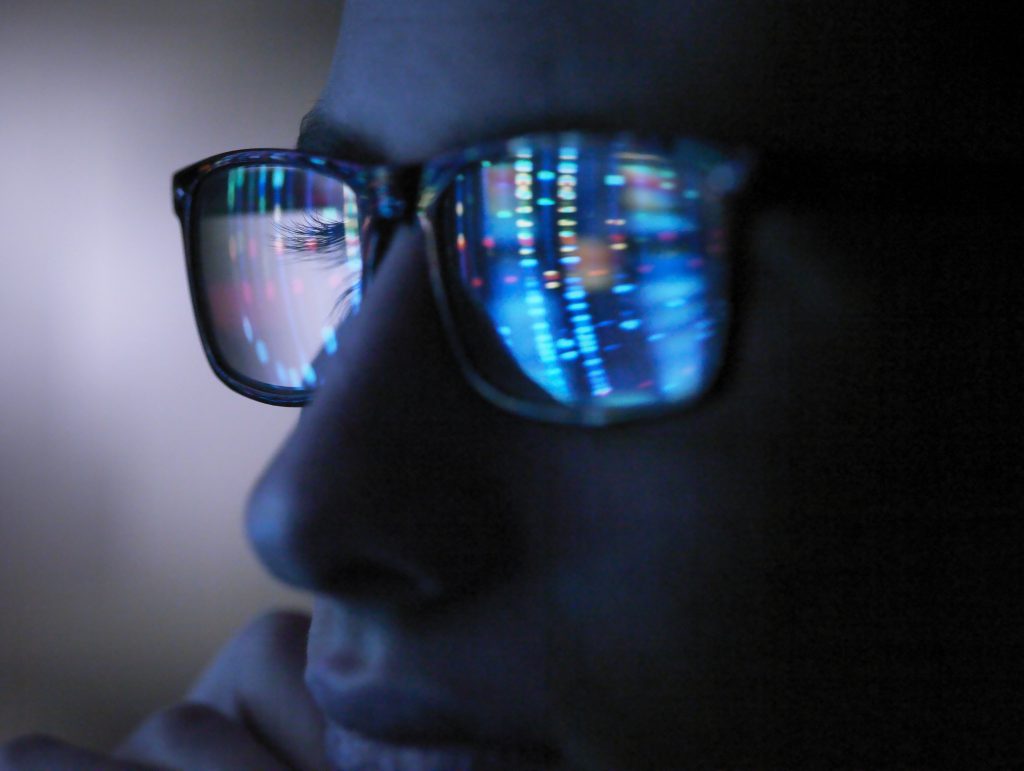
What is the alternative to A/B testing?
Ignoring the benefits of A/B testing, many companies still rely on what we’ve termed “A then B guessing”. This is when a new version of a page is created, is put online, and then its performance is measured without concurrent testing of the old version.
Imagine for a moment that you’re thinking of changing the background color of your landing page to boost conversions. To evaluate the effect of this change, you create two versions of the same landing page. One version is the original page with the present color. The other is the new selection.
Here’s what happens next if you’re following A/B test best practices. You’ll split your website traffic randomly and equally. One set of visitors will see the original version while the other views the modified version.
Now imagine making that same change but doing the following instead: instead of splitting your audience, you deploy the updated version to your entire audience.
How would you know whether any variations reported are the result of the color change? This method includes far too many variables to be successful. What if an increase in traffic is down to seasonality of your site, or a new ad campaign you started, or some recent press? By evaluating two versions simultaneously, you negate these effects.
What about A/A testing?
We should briefly mention another form of testing: A/A testing. A/A testing is similar in some ways to A/B testing. Both experiments call for the use of a “control group” and a “treatment group.” However, an A/A test has one significant difference: there is no disparity between the two testing scenarios. In essence, you’re testing the same thing against itself.
Validating the accuracy of A/B testing tools is the main reason why people run an A/A test. This type of analysis can also help to determine the baseline conversion rate and optimal sample size.
It can also ensure your tools are working as expected, though to save time, we’d recommend simply properly testing your page and tools during development. This ensures you don’t spend additional time on a test that doesn’t help you improve conversions.
Real world A/B test examples
The following examples from multinational companies can give you an idea of how the A/B testing process works.
Mercedes
Conventional wisdom says that mentioning price in a luxury ad is tacky. Mercedes used A/B testing to obtain a definitive, unbiased response. The company created three versions of a luxury ad campaign:
- One design didn’t mention price at all.
- Another featured it prominently.
- For the third version, the price had a peripheral placement.
For millennials, featuring price turned out to be the most important factor in considering the purchase of a luxury car.
Sony Europe
Sony Europe’s marketing team chose not to emphasize the personalization aspect of their customizable laptops in their banner ads. Their reasoning was that they expected it to be off-putting to customers. However, the click-through-rates of the new campaign were unimpressive.
To establish their customers’ preferences, the company created two new variants of the existing advertisement. One version stressed the personalization aspect, while the other advertised a promotion. They ran the three versions concurrently.
To their surprise, the test disproved their hypothesis. The personalization-focused ad was the best performer.
PepsiCo (a cautionary tale)
In 2009, PepsiCo took on a 35-million-dollar rebranding campaign for its orange juice brand Tropicana. The changes left the product unrecognizable to their existing customer base.
Gone was the image of a whole orange with a red and white striped straw sticking out of it. In its place was a clear glass of orange juice. What once stood out like a premium product now resembled a generic store brand.
Sales dropped by 20 percent, representing a 30-million-dollar loss nationally. Less than two months after its deployment, the old version was back. Between the rebrand and the restock, Pepsico spent over $50 million. Ouch!
For the record, it’s unclear whether the team behind the rebrand ran an A/B test before changing the design. However, the project’s outcome makes many advertisers feel that it was either overlooked or at best poorly executed.
Why you should run A/B testing
Today’s business environment is fast-paced and hyper-competitive. Companies wishing to thrive under these conditions must become adept at decision making. A McKinsey Global Survey found that organizations that excel at decision making make good decisions fast.
A/B testing tools can help you make data-driven decisions faster. Additionally, this type of test is cost-effective and time-efficient to execute. You also don’t need loads of traffic, so you’ll receive actionable insights faster.
Using this testing strategy, you can improve content engagement, reduce bounce rates, and achieve higher conversion rates and sales.
More data, more insights, more improvements. What have you learned today?
Keep the data coming and improve your customer experience now!